Appendix 4
The GGPM team
- Introduction
- Global Review
- Regional Outlook
- Country Performance
- Expert Consultations
- Ongoing applications and collaborations
- Statistical Tables
- Appendix 1 Summary of methods for the Green Growth Index
- Appendix 2 The international expert group
- Appendix 3 List of expert reviewers
- Appendix 4 The GGPM Team
- Partners
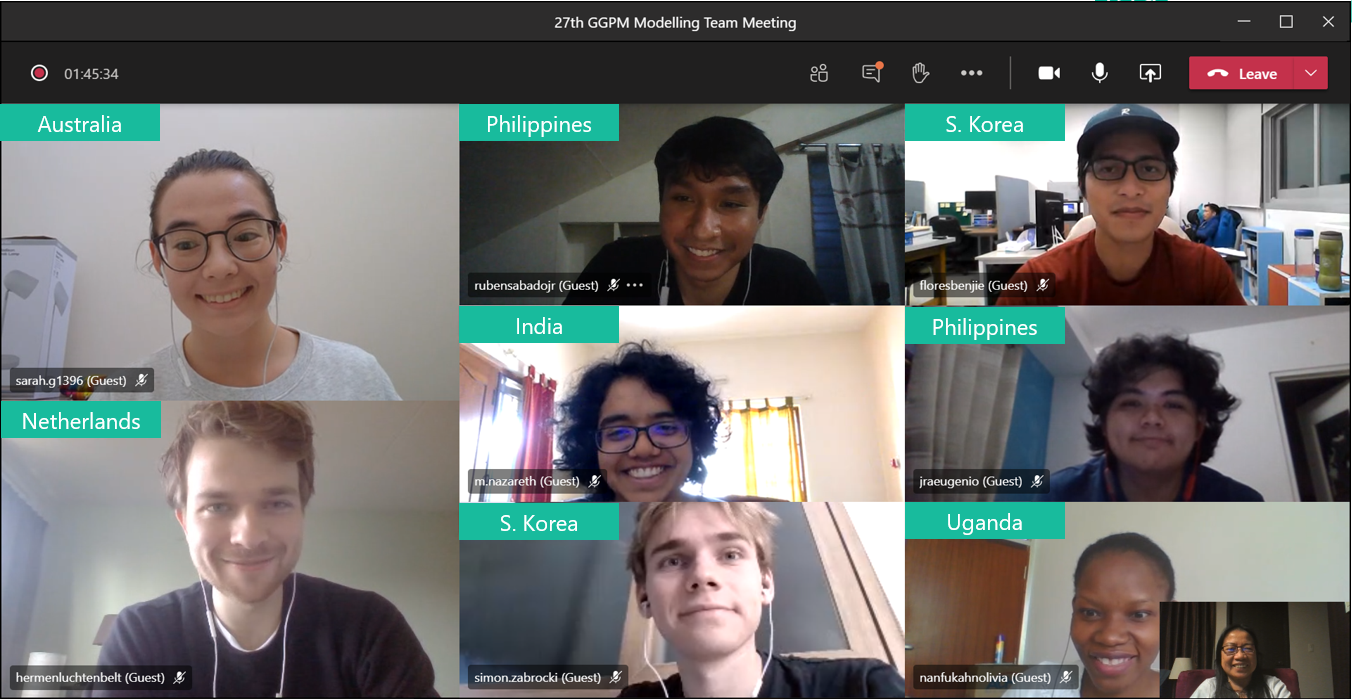
The GGPM team members during one of the online meetings from their respective work locations. From left to right and, from top to bottom: Sarah Gerrard, Hermen Gerrit Hendrik Luchtenbelt, Ruben Sabado, Jr., Michelle Nazareth, Simon Zabrocki, Benjemar Hope Flores, Jeremiah Ross Eugenio, Olivia Nanfuka, and Lilibeth Acosta.
Lilibeth Acosta is a Specialist in GGGI’s Climate Action and
Inclusive Development Division and Program Manager for the Green
Growth Performance Measurement. Lilibeth has over 15 years of
experience in indicator development, integrated assessment and
scenario modelling of climate change vulnerability and adaptation
as well as sustainable development in the fields of ecosystem
and biodiversity, agriculture and land use, and renewable energy.
She worked as development specialist in the National Economic
Development Authority in the Philippines, senior scientist in the
Potsdam Institute for Climate Impact Research in Germany, and
researcher in Environmental Science departments in the universities
in Japan, Belgium, UK and the Philippines. Before joining GGGI, she
worked as consultant in the ADB, UNCCD and UNCTAD. She holds
a PhD in Agricultural Policy from University of Bonn (Germany),
MPhil in Economics and Politics of Development from University of
Cambridge (England), and BSc in Agricultural Economics from the
University of the Philippines.
Jeremiah Ross Eugenio is a GGPM researcher and member of the
publication team of Sarena Grace Quiñones, who is coordinating
editorial, layout, and research support to the GGPM. He has
been part of Sarena’s team since October 2019. His tasks include literature review and preparation of references in Mendeley
software, encoding of results from the online survey and reviews of
online tools and literature, preparation of graphics for and analysis
of these results, and provide research support to the modelling
team of the Green Growth Index and Simulation Tool. He earned his
Bachelor of Science degree in Agricultural Economics with major
in marketing and prices from the University of the Philippines in
Los Baños. He participated in various seminars that are relevant
to the Index and Tool including farm tourism in the Philippines,
assessment of neighborhood and spillover effects of technical
efficiency of irrigated rice farms, and responding to food security and
inclusiveness concern in the ASEAN region.
Benjemar Hope Flores joined the GGPM as intern in 2018 and
consultant in 2019 supporting the preparation of the technical
report on Green Growth Index which was published in 2019. This
year, he is supporting the development of the Simulation Tool,
particularly the models related to efficient and sustainable energy
use. He works as teaching and graduate research assistant in the
Seoul National University of Science and Technology, where he is
currently doing his PhD study. He also worked as data collection
specialist in the private firm Rho AI, consultant in the Smart Communications, Inc., and as researcher in various institutions
including the University of the Philippines and Department of
Science and Technology, and Line Seiki Philippines Inc. He earned
his degrees on Bachelor of Science in Electrical Engineering
from the University of the Philippines in Diliman and Master of
Science in Electrical Engineering from Seoul National University
of Science and Technology.
Sarah Gerrard is a GGPM consultant for the 2020 Green
Growth Index and Simulation Tool. Her work in GGPM has
focused on results analysis for the 2020 Green Growth Index,
in particular a subregional analysis of green growth dimensions
as well as assessing top country performance. She has also
contributed as a leading author to the publication of the 2020
Green Growth Technical Report. Sarah has further been working
on the GGPM Simulation Tool, supporting the development of
the efficient and sustainable resource use and green economic
opportunities models. Before joining GGGI, she has previously
worked in sustainable urban development by interning at the
United Nations Economic and Social Commission for Asia and
the Pacific (UN ESCAP). Sarah holds a Master of Environmental
Science with a specialization in land and water management
and a BSc in Environmental Science and Natural Resource
Management, both from the University of Western Australia.
Hermen Luchtenbelt is a GGPM research consultant for
the 2020 Green Growth Index and Simulation Tool. He joined
the GGPM as an intern in May 2020 and as a consultant in
November 2020. His main contributions to the simulation tool
were with models related to natural capital protection, land-use,
and greenhouse gas emissions. Other tasks included preparing
the spatial maps in the 2020 Green Growth Technical Report.
Before joining GGGI, he did field work at the Osotua foundation
and supported in the development of a showcase for cattle,
culture, and wildlife interactions in the Masai Mara. Hermen has
a MSc in climate studies specialized in biogeochemical cycles
and a MSc in Environmental Economics and Natural Resource
Management at Wageningen University in the Netherlands.
Before that he completed his BSc in Economics and Governance,
specialized in Agricultural Economics at the same university.
Olivia Nanfuka joined GGPM team as intern in May 2020
and as consultant in the GGGI Country Office in Uganda in
November 2020. She is contributing to the development of
the Simulation Tool, particularly the models related to efficient
and sustainable energy use. Her key areas of interest include
improving access to modern energy; clean cooking energy and
reliable electricity for rural communities (bio-energy, hydro and
solar energy), energy efficiency and management, renewable
energy policy, energy economics, savings and energy yield
assessments. Before joining GGGI, she had experience working
as Health and Safety Assistant in the JUAJAMII start-up
company in Algeria as well as sustainability and policy intern in
the Atacama Consulting, shift superintendent in the Bwendero
Dairy Farm Limited Distillers, and teaching assistant in the
Ndejje University in Uganda. She completed her BSc Chemical
Engineering in the Ndejje University in Uganda and MSc Energy
Engineering in Pan African University Institute for Water and
Energy Science in Algeria.
Michelle Nazareth is a GGPM consultant for the 2020 Green
Growth Index and Simulation Tool, contributing to the analysis of
the results for the 2020 Green Growth Index and supporting the
development of the models related to social inclusion. She also
contributed to an article on social inclusion that was submitted
by GGGI to an international journal. She worked as intern in the
Environmental Synergies in Development and graduate research
consultant in the United Nations Office for the Coordination
of Humanitarian Affairs. She completed her Bachelor of Arts
(Triple Major) in Economics, Sociology and Psychology in the
Christ University in India and Master of Science in Development
Management in the London School of Economics and Political
Science.
Ruben Sabado, Jr. is a GGPM researcher and member of the
publication team of Sarena Grace Quiñones, who is coordinating
editorial, layout, and research support to the GGPM. He has
been part of Sarena’s team since July 2020. His tasks include
literature review and preparation of references in Mendeley
software, encoding of results from the online survey and reviews
of online tools and literature, preparation of graphics for and
analysis of these results, and provide research support to the
modelling team of the Simulation Tool. He earned his Bachelor
of Science degree in Agricultural Economics with major in
marketing and prices from the University of the Philippines in
Los Baños. He attended various seminars that are related to the
Green Growth Index and Simulation tool such as the Philippine
Rice Information System (PRISM) and success stories of the
Farmer-Scientists RDE Training Program (FSTP).
Simon Zabrocki joined GGGI as programmer and modeler
consultant in July 2020, with main tasks of developing an
automated collection and processing of data for the Green
Growth Index computation, designing user-friendly dashboards
to allow policy makers exploring and analyzing Green Growth
data and scores, and contributing to a policy simulation tool
development by implementing and integrating models and policy
scenarios. Before joining GGGI, he worked as data scientist in
HawaDawa company on air quality management in Germany
and in Sanofi, Biologics Development R&D in the United States,
Python developer for an applicant tracking system in Manatal in
Thailand, and teacher in Bac Ninh high School for gifted students
in Vietnam. He earned his Bachelor in Engineering and Master
of Science in Applied Mathematics in École Polytechnique
in France, and Master Mathematics for Data Science in the
Technische Universität München in Germany.